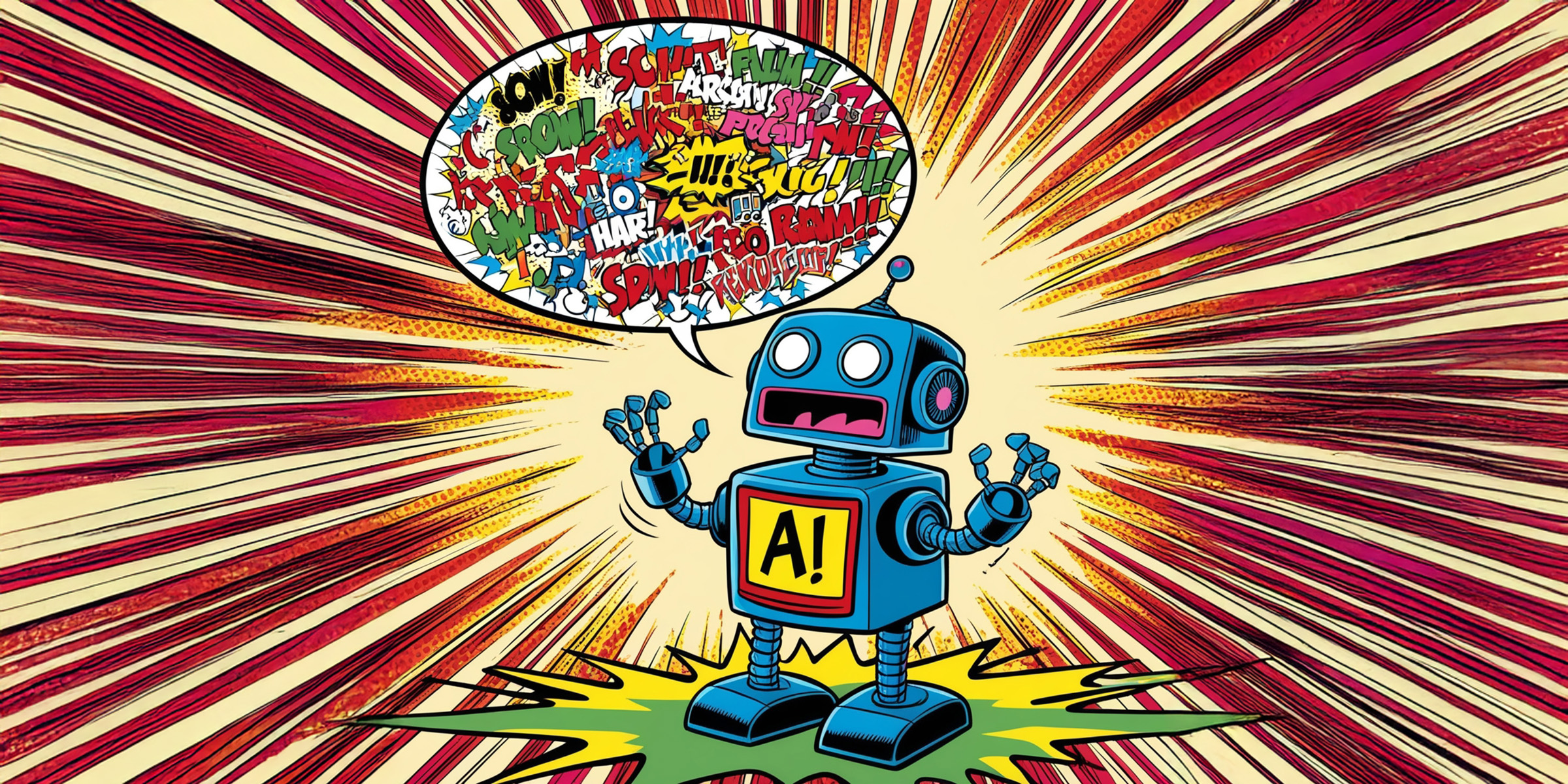
Who answers when AI misbehaves?
A new theory challenges how firms manage AI. Gudela Grote and colleagues published the paper in the Academy of Management Review. They argue that developers, users, and managers must share control and accountability through dialogue, negotiation, and careful design. The result could reshape how we trust AI.
- Read
- Number of comments
In a recent Academy of Management Review publication, Professor Gudela Grote and two colleagues address a dilemma that will soon trouble many firms. Advanced algorithms make decisions that often elude full human understanding. While corporate actors praise AI’s virtues, blame rarely settles neatly when these systems fail. If your AI discriminates against job candidates or serves up flawed financial forecasts, who stands behind it, and who should bear the weight of accountability?
The authors propose a theory of alignment between control and accountability. They argue that developers and users must share both, reshaping how organisations govern AI.
Blame game
Grote and her colleagues want to know how firms should handle questions of responsibility. Why is it fair to hold a human operator accountable for an outcome shaped by an inscrutable system that even its developers struggle to comprehend?
For decades, human factors research has shown that complex technologies can erode meaningful human control. Usually, managers purchase technology and hand it to employees. If something goes wrong, workers shoulder blame.
“In many organisations, there is a misalignment of who is in control and who is accountable. People are sometimes held accountable for something they don’t have any influence over, or people in control push away the accountability to somebody else,” explains Professor Grote, D-MTEC Professor of Work and Organisational Psychology. “That is why we’ve been arguing that accountability needs to go to the people that develop automated systems as they have much more control over the systems than users.”
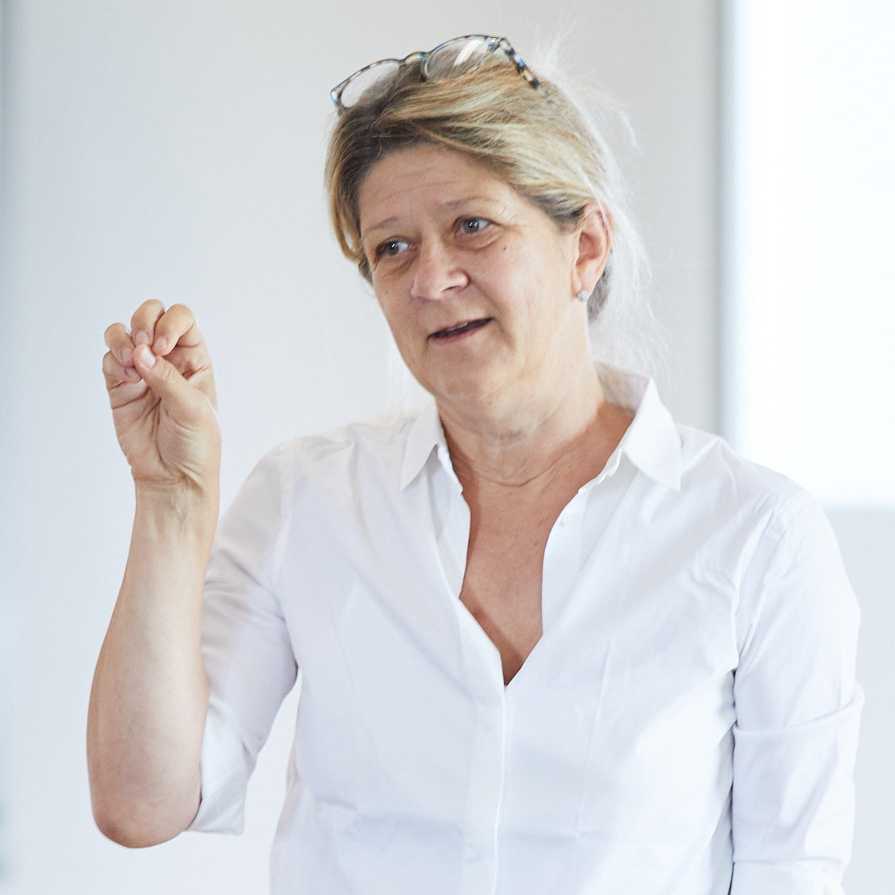
“Managing alignment of control and accountability with machine-learning-driven analytics tools, autonomous vehicles, and medical diagnostic systems is challenging as boundaries between system development and use begin to blur.”Gudela Grote![]()
Misalignments between control and accountability have been observed for a long time, for instance, in industrial control rooms and on flight decks. “Now, with machine-learning-driven analytics tools, autonomous vehicles, and medical diagnostic systems, managing these misalignments becomes even more challenging as boundaries between system development and use begin to blur,” says Grote. With advanced AI, even developers do not always know why the system made a particular decision. The problem grows thornier because these systems learn as they run. They adapt autonomously, thereby creating discrepancies between what the developer built and what the AI evolved into.
A new theory of alignment by sharing burden and power
The paper offers a theory to align control and accountability. Grote and her colleagues do not claim a neat blueprint. Instead, they present a conceptual framework for decentralised governance and joint negotiations. Their model encourages all stakeholders to meet, share perspectives, and define common norms.
Grote suggests that this involves more than cosmetic efforts. Firms must create forums where developers and users interact before deployment. Users need to understand how the technology operates and what influence they have. Developers, on the other hand, must appreciate the work environment that shapes how their creations will be used. Such dialogue can mitigate mismatches, foster trust, and clarify responsibilities.
In the corporate sphere, such adjustments are hardly trivial. Firms often celebrate the promise of AI to cut costs, boost productivity, and outsmart rivals. Yet fewer executives focus on the organisational ramifications of letting intelligent algorithms guide decisions. Grote: “Imagine an oncologist who gets told by an AI that a patient has cancer. Can they say ‘No, I don't think so’, or do they have to explain going against the AI-suggested treatment because there's more trust in the system than in the person?” As regulatory pressure grows, disregarding these questions may prove short-sighted.
Organisations must resist the temptation to let powerful suppliers dictate the terms. Grote wryly observes that in the world of Big Tech, decentralisation sounds naïve. But the cost of clinging to centralised control is growing. Public sentiment and legal frameworks are inching towards stricter demands. The hope is that firms will embrace collaborative governance to avoid more invasive regulation later.
Ad ex-ante!
For researchers, the new framework invites more empirical exploration. Academic literature often documents AI’s outcomes after deployment. The study nudges scholars to move upstream and engage with how AI is designed and implemented. The shift from retrospective analysis to proactive engagement could deepen understanding of how accountability emerges.
For practitioners, the implications are equally broad. Vendors and buyers of AI must rethink how they set up contracts and training. They must clarify who manages data quality, who decides how much complexity is tolerable, and who assumes liability. This is especially urgent in high-skate fields like healthcare, where doctors might rely on AI-driven diagnoses they cannot fully interpret.
Without a framework for shared control and accountability, trust will erode. Professionals will hesitate to rely on automated assistants, knowing that if something goes wrong, they will be blamed.
The authors’ emphasis on integrative negotiation hints at a future where AI deployment resembles a policy exercise as much as a technical endeavour. Teams must convene early and often, expose their assumptions, and exchange insights. This might mean that software developers talk to human factors experts, user representatives, and regulators. They might choose simpler, intelligible models rather than chasing the latest black-box innovation. They might learn from each other’s constraints, producing technology that works in the messy reality of corporate life.
As public scrutiny grows and regulators start looking closer, firms investing in shared accountability will gain a subtle advantage. They will show that they know how to “tame” AI not by subduing it with force but by adjusting their structures, responsibilities, and collective mindset.