Machine Learning for Industrial Applications
Register for the upcoming D-MTEC Short Course and gain access to machine learning methods in industrial applications, addressing challenges like scarce labelled data, highly variable operating conditions and diverse system configurations.
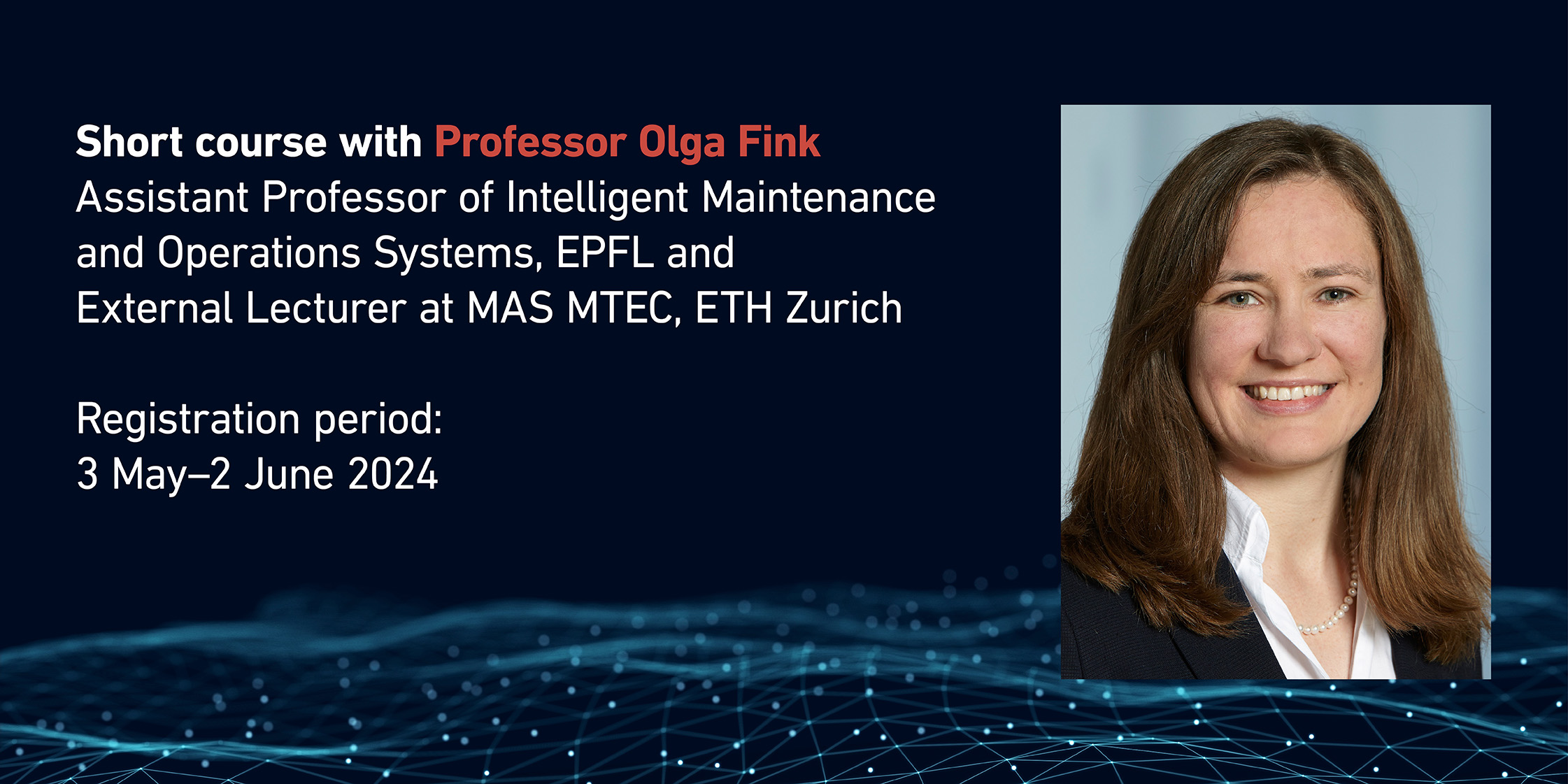
This course explores ML model development and (hybrid) digital twin concepts within complex environments, integrating practical exercises, case studies, and insights from industry experts to offer an in-depth exploration of how machine learning techniques can be applied to solve real-world industrial challenges.
Participants will learn how to select, design, optimise and evaluate machine-learning algorithms to overcome the challenges faced by intelligent maintenance and operation systems in complex engineered systems.
Practical exercises will illustrate the application of specific algorithms, while group discussions will delve into case studies to develop comprehensive understanding. The course will be enhanced with contributions from several guest speakers from industry who will offer insights into real-world applications and detailed case studies.
This course will take place together with MAS MTEC students and will give participants the opportunity to expand their network and be part of the ETH community.
Participants will be able to
- understand the main challenges faced by intelligent maintenance and operations systems
- learn to select appropriate tools for specific problems
- learn to evaluate the performance of applied algorithms
- learn to identify the requirements for the deployment of algorithms
- learn to identify potential misconceptions and fields of improvement
- learn how to define a problem is a way that allows for its solution
- Introduction to condition monitoring and predictive maintenance systems
- Introduction to the concepts of digital twins and their potential fields of application
- Feature extraction and selection methodology
- Machine learning algorithms for fault detection, isolation and prognostics
- End-to-end learning architectures (including feature learning) for fault detection and fault isolation
- Unsupervised and semi-supervised learning algorithms for predictive maintenance
- Performance evaluation
- Predictive maintenance systems at fleet level
- Domain adaptation and generalisation for fault detection and diagnostics
Lecturer
Professor Olga Fink
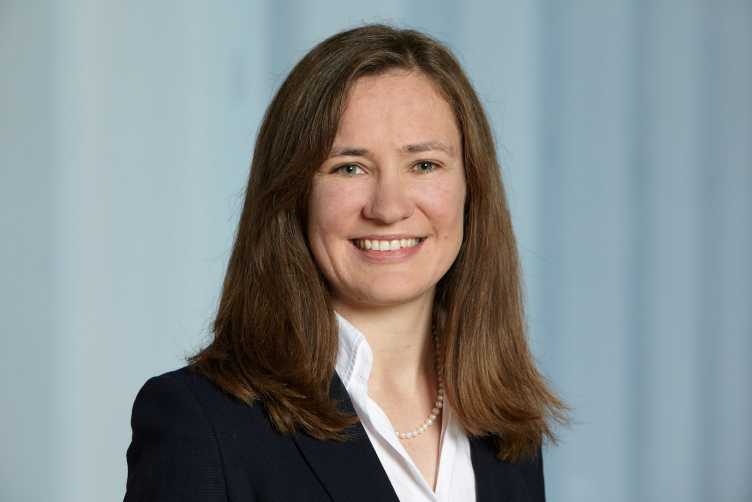
- Assistant Professor of Intelligent Maintenance and Operations Systems | EPFL
- External Lecturer MAS MTEC | ETH Zurich
- external pageLinkedIn Profilecall_made
Contact
ETH Zurich
D-MTEC
Weinbergstrasse 56/58
8092
Zurich
Switzerland